We propose a novel approach where a proxy model combines limited information about a spatial configuration of the hydrocarbon field and a simplified description of the governing physics. The approach contains several stages: in the first, the spatial connectivity graph is generated based on positions of wells and a limited log interpretation. Next, unstructured gridding is applied for the construction of spatial connectivity graph. The governing physical description is integrated using Operator-Based Linearization (OBL) approach. The parameters of this proxy model are regressed to the observation data using gradient optimization assisted by machine learning.
The proposed approach is validated based on high-resolution Brugge-field model18. The synthetic production data for the training period was generated based on high-fidelity model run with varying BHP controls following the original Brugge model schedule with a 5% white noise imposed to resulting oil and water rates. The discretized model for high-fidelity Brugge-field and proxy model is shown in Fig.18. Here, the number of control volumes decreased by more than two orders of magnitude.
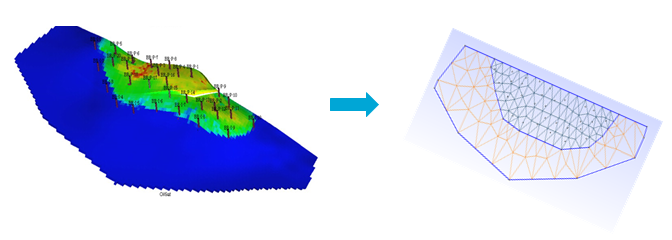
The resulting proxy model performs three orders of magnitude faster when applied in production optimization in comparison to the high-fidelity model (0.03 vs. 97.3 sec). Fig.19 illustrates a two-year production forecast for high-fidelity and trained proxy model which indicate relatively accurate rate prediction for all 20 production wells. Notice that in the case of data-driven models, underlying geological information was never used.
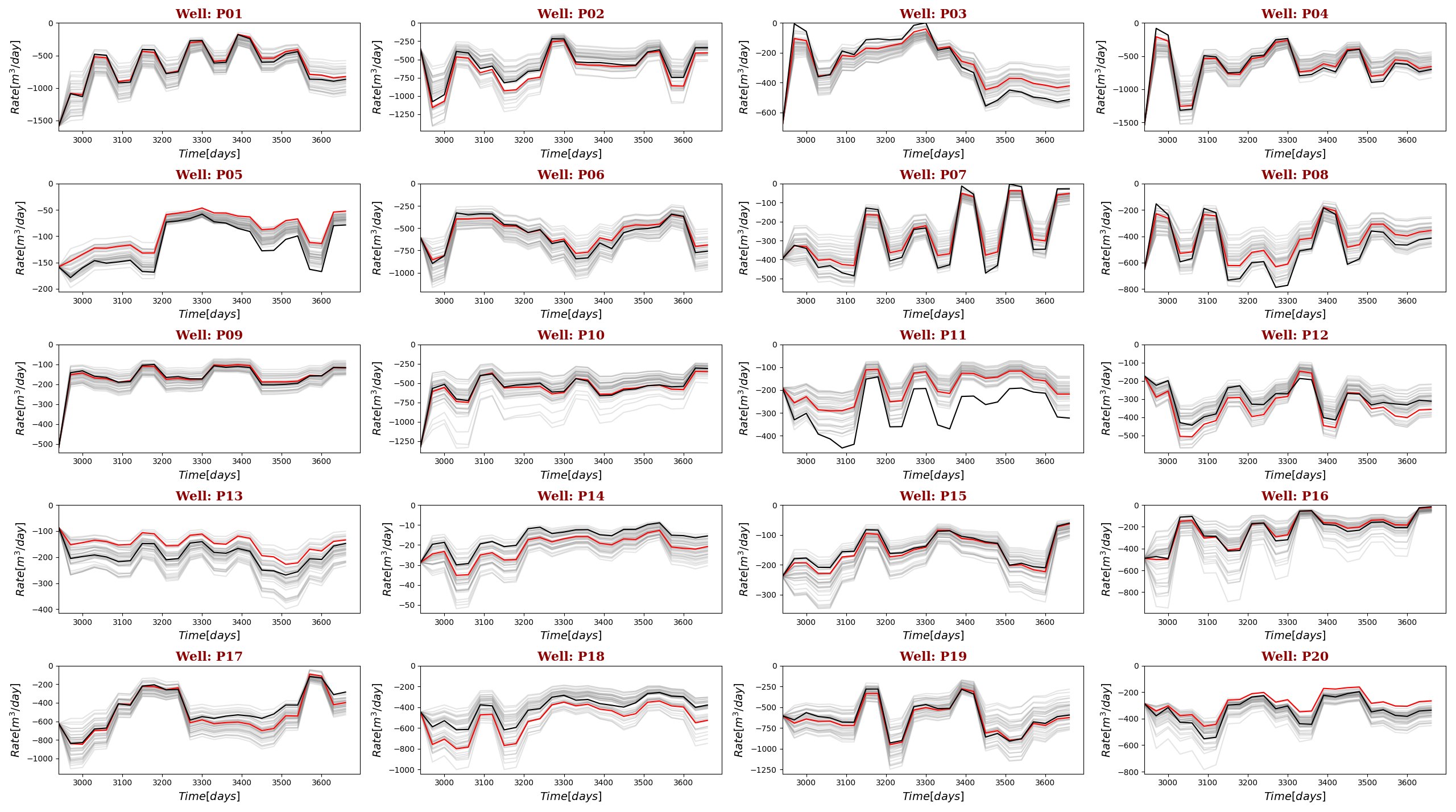